|
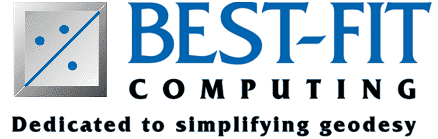 |
|
Network Adjustment FAQs
Back to FAQs
Why should I perform minimally constrained adjustments (one station
fixed), before performing fully constrained adjustments (two or more
stations fixed)?
A minimally constrained adjustment is performed in 1D, 2D or 3D
space. Because the network is only tied to one ground position, it can freely
move during adjustment. Any measurement blunders on your part are therefore more likely to be isolated and detected (provided good network geometry has been
established).
On the other hand, once a network is constrained to two or
more control stations, it is forced to accommodate itself, plus the
previously established control. This introduces errors from
prior surveys into your network, making it more difficult to locate any
errors in your field work. Ideally, a constrained adjustment should not
be performed until all obvious outliers have been detected and removed or
remeasured. Care should always be taken when selecting reliable control
stations for the constrained adjustment.
Since GPS baselines are based on the WGS 84 datum, how can I use them
on my country's local datum?
GPS baselines are vectors in 3D space (dx, dy, dz). Their orientation is
based on the WGS 84 datum. During a constrained adjustment, the least
squares process will automatically attempt to warp them to fit the local
control stations (based on your local datum). This may cause some baselines to appear as outliers, when in
fact they are not. A better way is to rotate and scale each vector
according to the local control - then apply least squares. COLUMBUS
allows you to enable automatic rotation and scaling of GPS baselines via
switches in the OPTIONS - NETWORK OPTIONS - NETWORK SETTINGS dialog box.
During each iteration of the adjustment, rotation and scaling corrections
are applied as the adjustment proceeds.
How can I fix the latitude or longitude of a station (but not both)?
Use the geodetic coordinate observation. To fix the latitude, set the latitude covariance (NN) element
to be small (e.g., 0.00000001m). Set the longitude covariance element (EE)
deviation to be large (e.g., 10m). This will allow the longitude the
freedom to move, but the latitude will be held virtually fixed. Allow the station, with the same name, to float during the nework adjustment.
Can I combine terrestrial measurements (observations) with GPS and obtain
high-quality results?
Terrestrial observations can be as good or better than GPS. One of the
greatest advantages of GPS is no traversing is required. The farther you
must traverse in a terrestrial survey, the greater the likelihood for errors
to accumulate in your network. However, if you were to measure a network
using GPS or terrestrial observations in which no traversing was required,
you would soon discover the terrestrial network could be as good or
better than the GPS network. Therefore, you should freely mix GPS and
terrestrial measurements as needed. When using terrestrial measurements,
be sure to always measure the instrument and target heights at each setup.
Also, you may want to experiment with
the internal refraction correction or supply zenith angles which already
have a built-in correction applied by you.
What is the purpose of the Chi Square Test On Variance Factor? Where does the number generated come from?
The Chi Square Test On Variance Factor is used to test the hypothesis that the a priori variances (standard deviation squared) for all the observations in the network as a whole are realistic based on the adjustment.
If the a priori variances for all the observations as a whole are in agreement with the adjustment, the A posteriori Variance factor will approach 1.0. If the interval generated (low end below 1.0, high end above 1.0) contains 1.0, then the A Priori Variance factor is said to be statistically close enough to 1.0 to consider it to be 1.0.
For example: If you have 36 GPS observations in your network (12 delta X, Y and Z) and you say they are good to within +-0.005 meters, then we would expect them to not be adjusted by more than this amount. Indeed, if all 36 observations were good to within +-0.005 meters, they would not be adjusted by more than +-0.005 meters (assuming a free adjustment in which you are not including any errors from prior surveys). However, if on average they were adjusted by +-0.010 meters, then we might suspect a problem with our initial estimates or a possible blunder (measurement error in one or more observations) exists. In this case, the A Priori Variance factor would be greater than 1.0. Whether or not it is significantly greater than 1.0 is determined via the Chi Square Test. Alternatively, on average, if each observation were adjusted by only 0.002 meters, then the A Posteriori Variance Factor would be less than 1.0. Again, whether or not it is significantly less than 1.0 is determined by the Chi Square Test.
Why should I be concerned if the a posteriori variance factor is statistically different from 1.0 after performing a network adjustment? If I set the
A Priori Network Variance scaler in the OPTIONS - NETWORK OPTIONS - SCALERS dialog
box to the a posteriori variance factor, then readjust, the new a posteriori variance factor goes to 1.0.
The a posteriori variance factor (APVF) is an indicator (for the network
as a whole) as to the consistency of the adjusted network, given the
a priori predicted errors. The predicted errors are represented by each observation's
standard deviation or, in the case of GPS baselines, by the variance
covariance matrix for each baseline. If on average, each observation is
adjusted by an amount larger than its predicted error, the APVF will tend
to be greater than 1.0. If on average, each observation is adjusted by
an amount less than its predicted error, the APVF will tend to be less
than 1.0. In either case, you may not be correctly estimating the
quality of your measured observations, or you may have a few bad measurements
in your survey (those receiving large adjustment). If the latter is the
case, you should attempt to isolate the bad observations and possibly
re-measure them, since they may be distorting your results.
What is the difference between a Fixed station and a Constrained station?
A fixed station is simply a constrained station which is not allowed to move during an adjustment. In fact, the user could select constrained stations to be fixed stations in the network adjustment if he were to set each station's coordinate component standard deviations to a very small value (i.e., latitude standard deviation, longitude standard deviation, height standard deviation to a value such as 0.0000001 meters).
To eliminate this step, COLUMBUS provides the fixed station selection list, which in effect does this automatically during a network adjustment.
If, however, the user wanted to allow a control station the possibility to move during a network adjustment (rarely the case, but some folks insist on it), he would select the station to be constrained. The amount of movement would be constrained (not necessarily confined) by the standard deviation for each coordinate component.
For example: Suppose Joe Surveyor has several control stations in his network. Suppose further that for one of these stations he would like to allow movement during the adjustment. He could just set the station to be free, but it may move more than he wants. Alternatively, he can set the station to be constrained in 2D (latitude and longitude). By specifying a known coordinate and standard deviation for both latitude and longitude, Joe Surveyor is applying a constraint on that station. The station will be allowed to move, but will be constrained by these standard deviations. During the adjustment, the coordinate components for this station may move a bit more or less than the governing constraint. They may not move at all if the known coordinate components for the station are accurate and the survey is of high quality.
Most users do not use the constrained option, because they want their control to remain fixed without having to explicitly define small standard deviations for each fixed station component (latitude, longitude and height).
Some users use the constrained station in order to propagate the statistics (standard deviations of the adjusted coordinates) from a prior survey (in which those stations are now the control in the new survey). Others would consider those stations adjusted and good, and should be fixed for any subsequent networks tied to them.
Please see the FAQ How can I fix the latitude or longitude of a station (but not both) for the preferred way to constrain a station using a coordinate observation.
What is the purpose of the Confidence Level setting? What is the difference between a Confidence Level setting of 0.95 and other values?
The confidence level parameter allows the user to set up the Chi Square probability scaler useful for making hypothesis tests as to the quality of the survey. For many projects, the purchaser of the survey wants to know how good various statistics about the survey are at the 95% confidence level. COLUMBUS can be set to any confidence level between 0.001 and 0.999 (0.1% to 99.9%).
The least squares process automatically computes all statistical results at the 68.3% confidence level (i.e., 1 sigma). This is why the results generated are in terms of 1 standard deviation.
Many of the statistical results generated during the network adjustment give the surveyor an indicator as to the quality of the survey (or portions of the survey).
For example: The distance error is an indicator as to the quality of the adjusted distance between two stations. If the confidence level were set to 68.3% (1 sigma), we could infer that the true chord distance between any two stations was equal to the computed value (adjusted) +- its standard deviation. If we were to remeasure this distance hundreds of times, we would expect each measured distance to be in the range of the adjusted distance +- its standard deviation 68.3% of the time. Put another way, at the 68.3% confidence level, we expect the true distance between the two stations to fall somewhere between the adjusted distance +- its standard deviation.
As is often the case, the surveyor (or their customer) wants to know this type of information at the 95% confidence level. To report this, the 1 sigma results must be scaled to roughly 2 sigma. That is, the standard deviation must be increased to 95% confidence that the true value lies somewhere between the adjusted value and its scaled standard deviation.
Most surveys are adjusted at the 95% confidence level. Try changing the number to 68.3% and see what happens to the distance errors. They will become more optimistic (lower PPM's and higher ratios). You can also change it without re-adjusting. This can save considerable time when processing with large surveys.
Within the Residual Distribution histogram, what are the units for the Y-axis? What are the meaning of the values 0.1, 0.2, 0.3...?
The units along the Y axis refer to the relative frequency of occurrence for the standardized residuals. If the standardized residuals are distributed normally (as represented by the bell shaped curve), we can expect the probability of any one standardized residual to fall within a class (intervals along the X axis) to be equal to the class size (0.5 standardized residuals in COLUMBUS) multiplied by the relative frequency of occurrence. This is represented by the area under the curve for each class interval.
The sum total of area under the curve is 1.0 (0.5 on each side of the mean). The sum total of area under the curve spanning -1 sigma to +1 sigma is roughly 0.68. From -2 sigma to +2 sigma is roughly 0.95, -3 sigma to +3 sigma is 0.99, etc.
If the standardized residuals are normally distributed, we would expect 68.3% of them to fall between the interval -1 sigma and +1 sigma. We would expect 95% of them to fall within the interval -2 sigma to +2 sigma. If 80% of them fall within +- 1 sigma, the standardized residuals are not normally distributed, even though it may be more desirable.
What are Height Confidence Intervals?
The Height Confidence Intervals are the standard deviation between two stations if the adjustment is computed at a confidence level of 1 Sigma (approx 68.3% --> 0.683) and you are examining the relative height confidence intervals. If the adjustment was processed at 95% (0.95), the computed standard deviations are scaled to a larger value, so that we can say with 95% confidence that the true height difference between the stations is somewhere between the adjusted height difference +- the scaled standard deviation.
The smaller the scaled standard deviations, the better the results. If you change the Confidence Level to 0.683, you will notice the 1D expansion factor becomes almost 1.0. Hence, no real scale change is applied, but then we can only make the above statement with 68.3% confidence.
The 2D expansion factor applies to error ellipses (a two-dimensional result), while the 3D expansion factor applies to error ellipsoids (a three-dimensional result).
The expansion factor is tied to the number of variables. For the error ellipse, there are X and Y (or East and North) components. Since there are two variables, we use a 2D expansion factor. For the error ellipsoid, there are X, Y and Z components (East, North and Up).
What are "mark-to-mark" reductions?
Mark-to-mark reductions are corrections applied to the field observations before the adjustment. Mark-to-mark refers to reducing the field observations to the monument level at the AT and TO station. Terrestrial observations are rarely measured mark-to-mark. The instrument and target heights are commonly set above the monument (or a location on the ground which may not yet be monumented), but they could also be below the monument. Knowing the instrument and target heights, field observations can be corrected to monument or ground level.
For station pairs (AT and TO stations) which are very close together (a few hundred feet), the mark-to-mark reduction computation is trivial. However, for stations farther apart, other factors come into play - such as curvature of the earth and the height of each station. Observations most affected include chord distances and zenith angles.
GPS observations are usually provided at mark-to-mark. There usually is no instrument and target height attribute for GPS observations. The post-processing software handles the instrument and target heights (internaly), before delivering the final vector.
What is the difference between a corrected observation and an adjusted observation?
Corrected observations are only applicable to terrestrial observations. There are four primary corrections applied by COLUMBUS before the adjustment begins:
- Mark-to-mark reductions, as described above.
- Refraction corrections to zenith angles (optionally set up in the Options module).
- Corrections due to deflections of the vertical to convert astronomic observations to geodetic observations.
- Average bearing rotation to true azimuths.
Other corrections are applied (optionally by COLUMBUS) during the adjustment, such as the scaling of linear observations and the Rotation and Scaling of GPS observations.
Adjusted observations are the adjusted corrected observations. These are the observations (which when adjusted) result in the best solution for the entire network of adjusted stations.
Sometimes I am unable to change Options Settings in COLUMBUS; why?
In order to change certain fields in the OPTIONS area, you need to clear the current adjustment. Changing these options while the network adjustment views are active could lead to incorrect interpretation of the results, so we disable these fields.
Some fields can be changed (e.g., the Linear Units).
Network Adjustment and Coordinate Transformation Software Solutions Since 1990
info@bestfit.com Ph 503-531-8819
Copyright © 1995-2011 Best-Fit Computing, Inc. All rights reserved.
|
|
|